Machine Learning Workshop
29 March – 1 April 2022
Sophisticated machine learning techniques are moving towards operational use. This workshop will discuss applications for numerical weather prediction and climate services.
Learn more about the four sessions below.
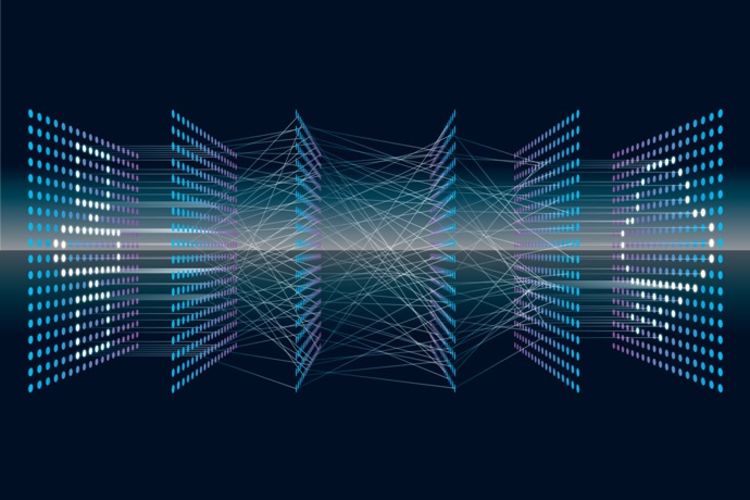
Tuesday 29 March
Machine learning for emulation of model components
Chair: Matthew Chantry
Tom Beucler
University of Lausanne
Physics-guided and causally-informed machine learning for climate modelling
By incorporating thermodynamics into machine learning models of storms and clouds, we can help the models generalise across a wide range of climates. But what if we lack the relevant physical knowledge for generalisation?
By selecting the most informative predictors via causal discovery we can alleviate the generalisation and stability problems that limit the potential of machine learning for climate modelling.
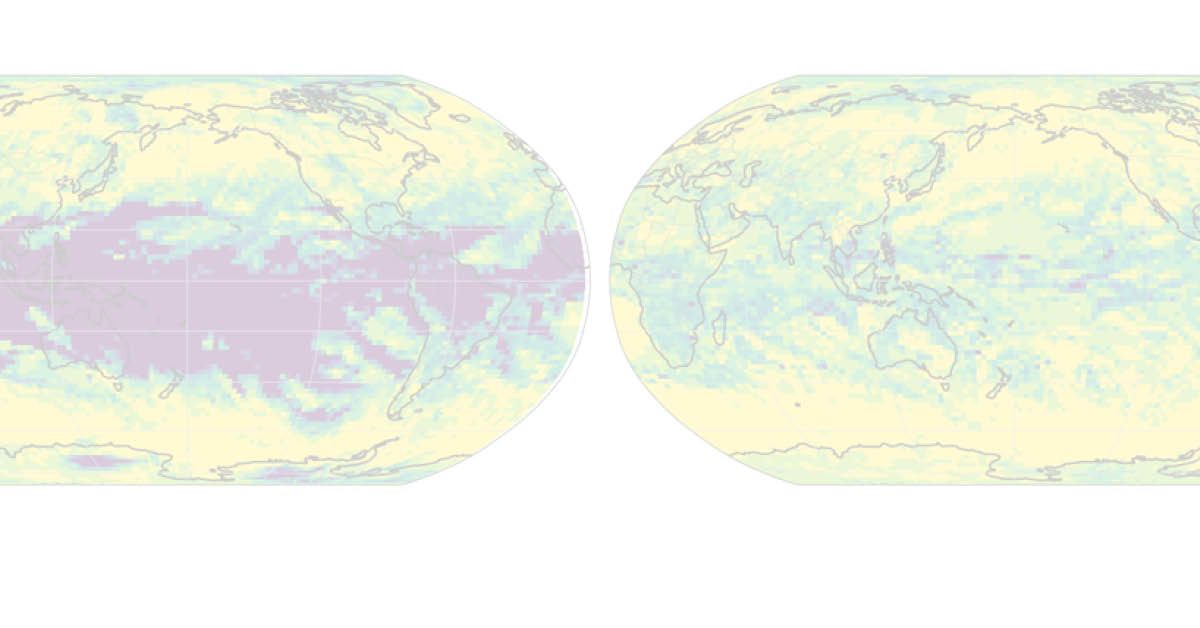
Paula Harder
Fraunhofer Institute ITWM
Physics-informed learning of aerosol microphysics
Despite their importance for climate processes, aerosols are often not modelled in detail in global models, due to high computational costs.
To tackle that problem, we train a fast physics-constrained neural network to emulate an aerosol microphysics model, achieving sufficient accuracy and a speed-up of 64x.
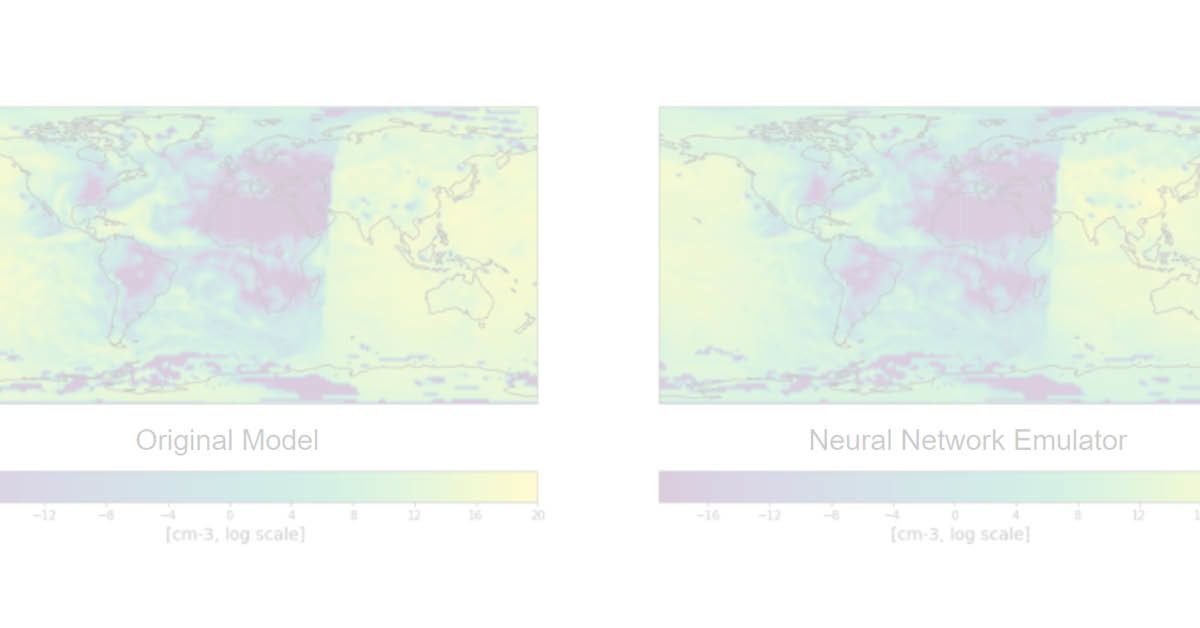
Wednesday 30 March
Machine learning for forecasts from now-casting to seasonal
Chairs: Peter Dueben, Shraddha Gupta and Nikolaos Mastrantonas
Petrina Papazek
ZAMG
Solar power forecasts using deep learning
Fostering solar power as a sustainable energy source demands accurate, location-optimised, and highly resolved forecasts of power production.
Deep learning enables us to consider data from historical time series to model highly variable changes in expected power production and so optimise forecasts based on machine learning.
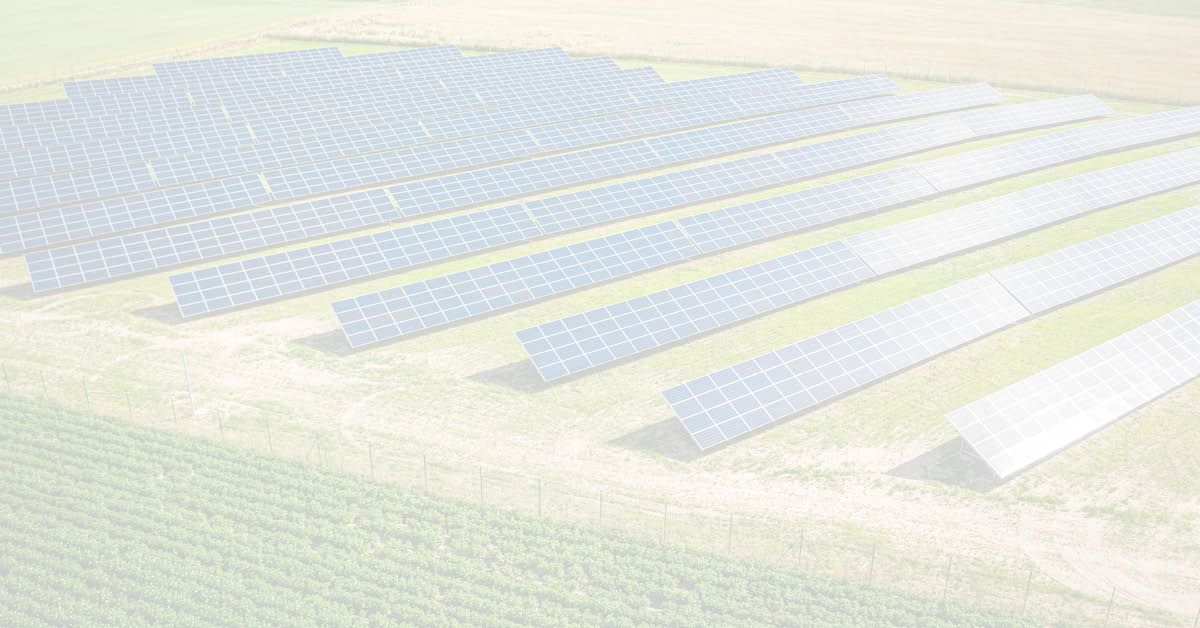
David Landry
Computer Research Institute of Montreal
Choosing the best seasonal forecasting model using neural networks
As part of an international prize challenge to apply artificial intelligence to weather data, we combine established and well-known methods with newer neural networks to improve seasonal forecasts.
These long-range forecasts have applications in critical areas such as renewable energy production, natural resource management, and climate resilience in general.
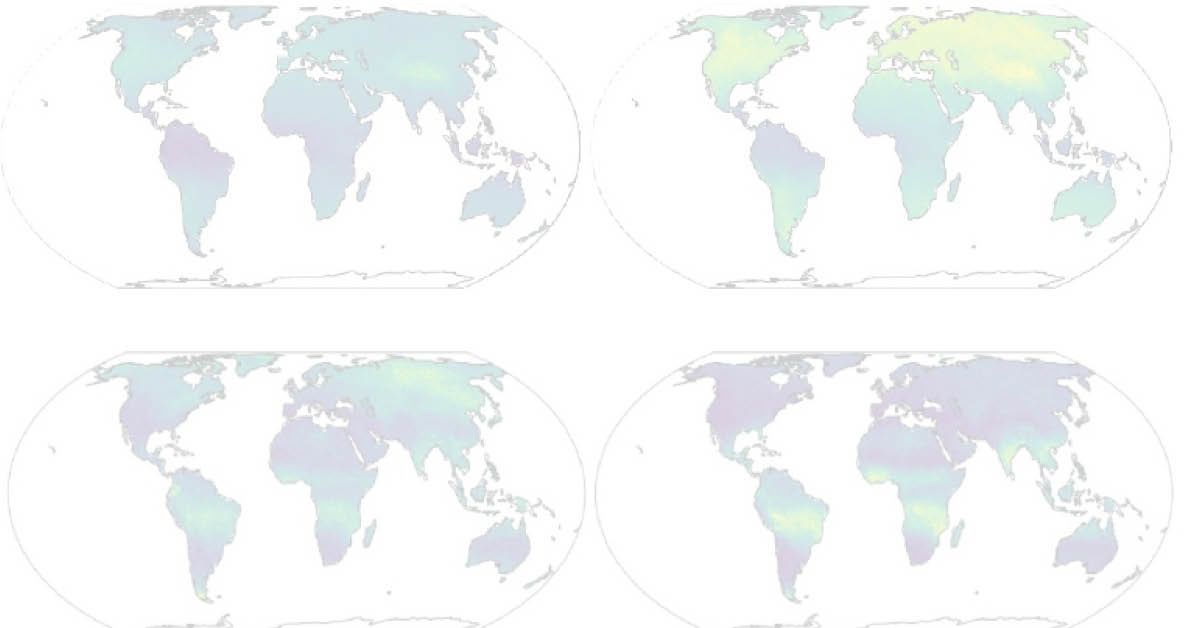
Dale Durran
University of Washington, Seattle
Deep learning weather prediction: new scientific horizons
Deep-learning-based weather prediction (DLWP) models can make data-driven predictions for the evolution of our atmosphere in ways that go beyond our current physics-based understanding. What new horizons can this, and other unique properties of DLWP, open for scientific exploration?
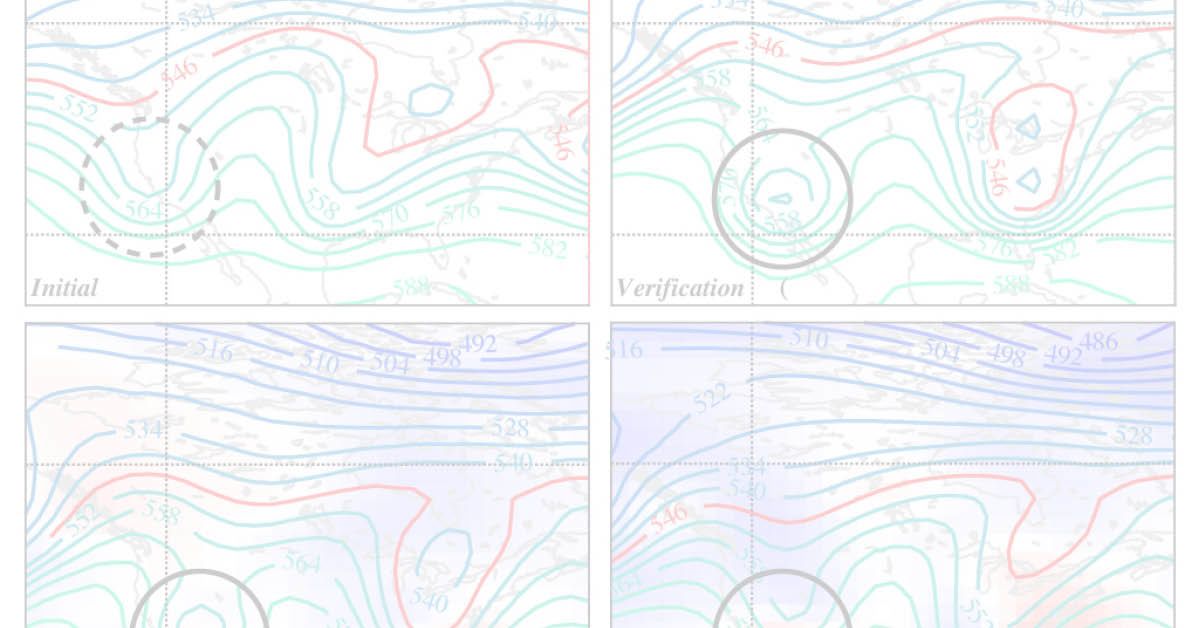
Thursday 31 March
Machine learning for feature detection and user applications
Chairs: Mihai Alexe and Jesper Dramsch
Arnaud Mounier
Météo-France
Using a neural network to explore rainfall patterns
Extracting and conveying relevant information from ensemble forecasts can be challenging, but is vital for making accurate predictions about weather.
We introduce an approach that uses a neural network to extract information on rainfall patterns and check for similarities with previous events and so define predictability of individual events.
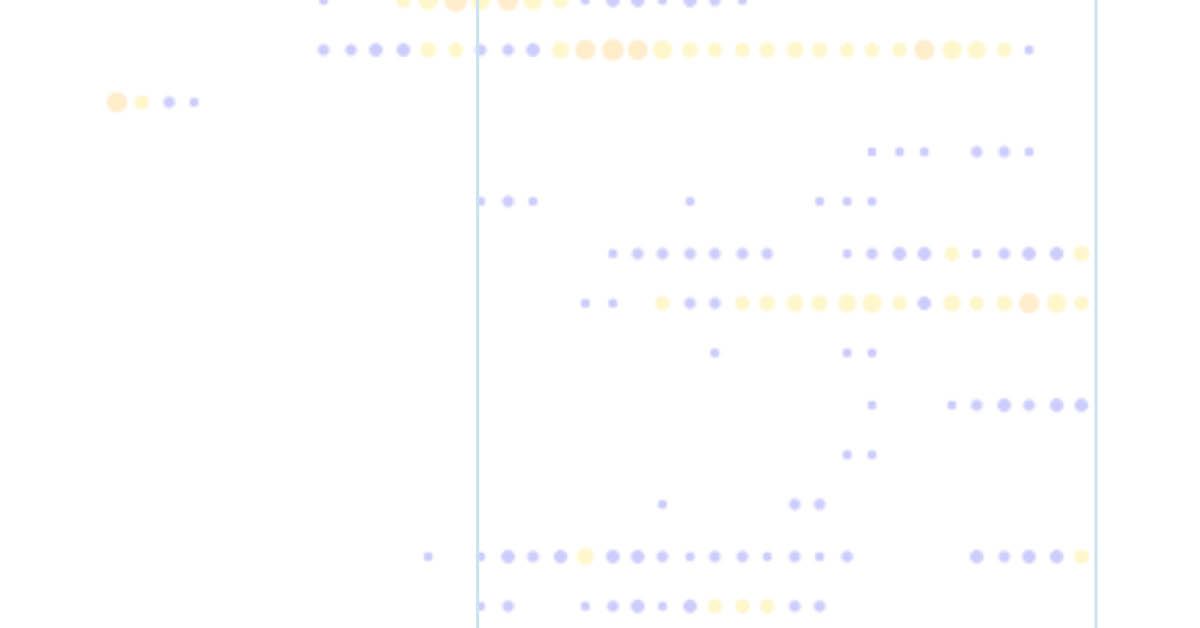
Clara Betancourt
Jülich Supercomputing Centre
How ozone research can benefit from explainable machine learning
Machine learning approaches are widely applied to predict the spatial variability of tropospheric ozone.
We show how the reliability and generalisability of machine learning models for ozone prediction can be evaluated by analysing their latent spaces and feature spaces. This allows us to gain new insights across the global domain.
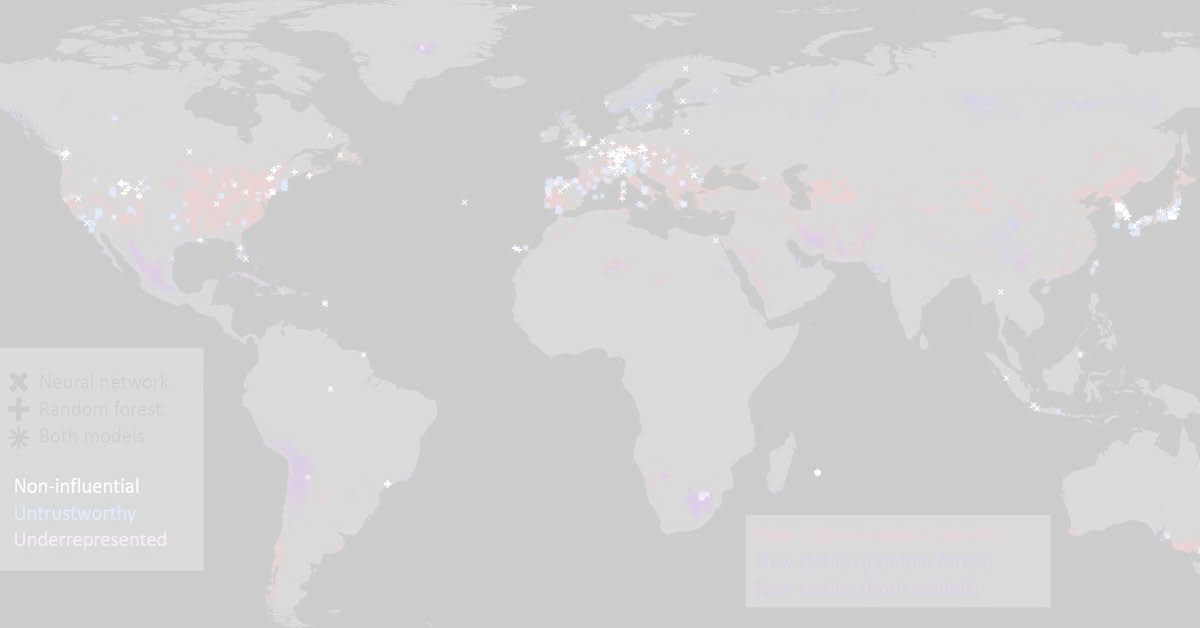
Friday 1 April
Machine learning tools and high-performance computing
Chairs: Peter Dueben & Florian Pinault
Peter Bauer
ECMWF
The European Union Destination Earth project and its potential for boosting machine learning
Digital twins will advance how we create and use information that helps us better adapt to climate change and manage the risks of environmental extremes.
Machine learning will be key to achieving the speed and versatility that is needed for the unique level of interactivity these enhanced models of Earth will provide.
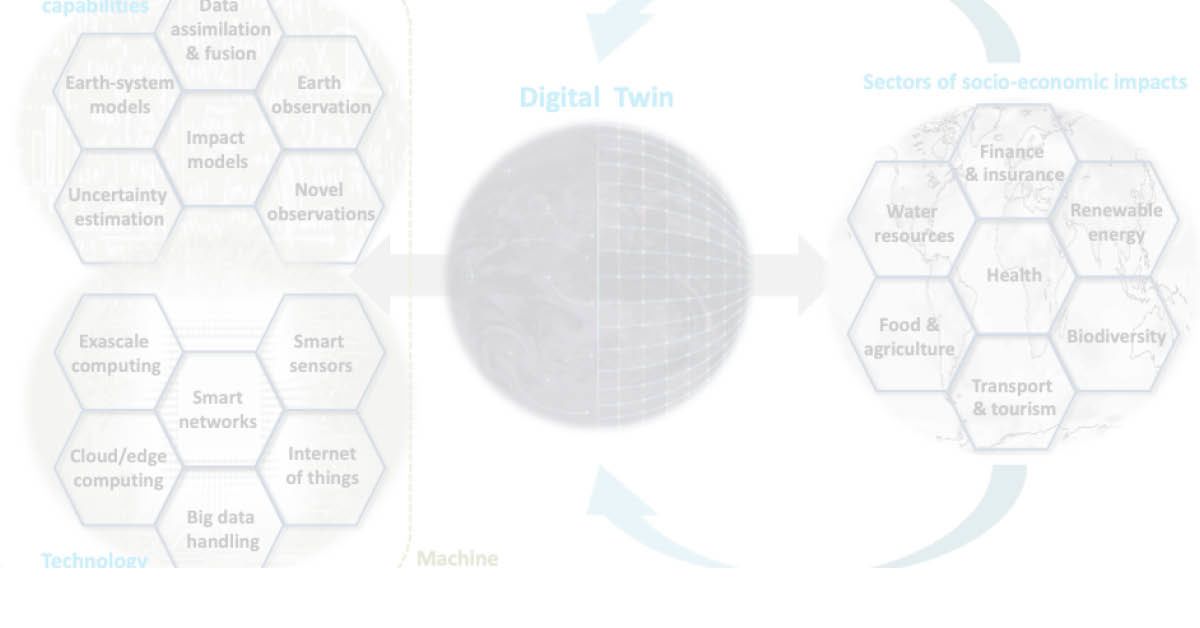
Bianca Zadrozny
IBM Research
Generative machine learning for extreme climate scenarios
There is growing need for plausible ‘what-if’ climate scenarios to help users understand exposure to extreme events, and examine resilience and mitigation strategies.
Machine learning techniques offer a pathway for generating climate scenarios and can improve the characterisation of ‘grey swan’ events; those with a low likelihood of happening, but a potentially large impact.
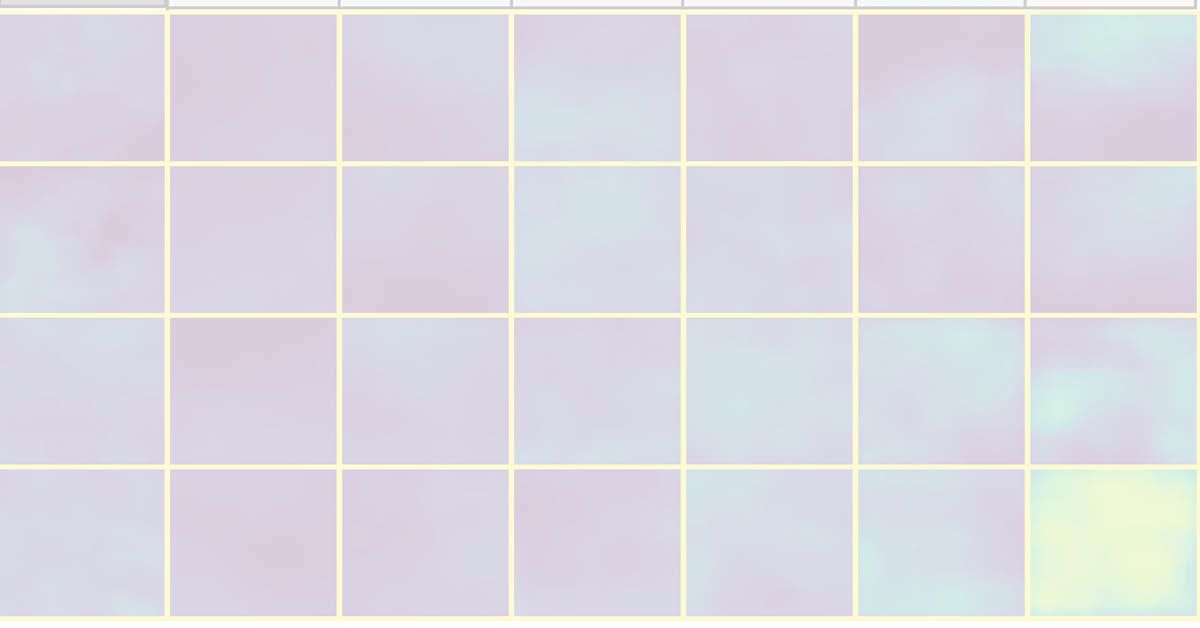
All the sessions are available to watch again via the event webpage.
We have an extensive education programme to support Member States and Co-operating States in the training of scientists in numerical weather forecasting, and in making use of our forecast products.
Find out more at ecmwf.int/en/learning