Machine Learning
at ECMWF
ECMWF is opening new job positions to support using machine learning within the European Union's Destination Earth initiative
Scroll down to learn more about machine learning at ECMWF
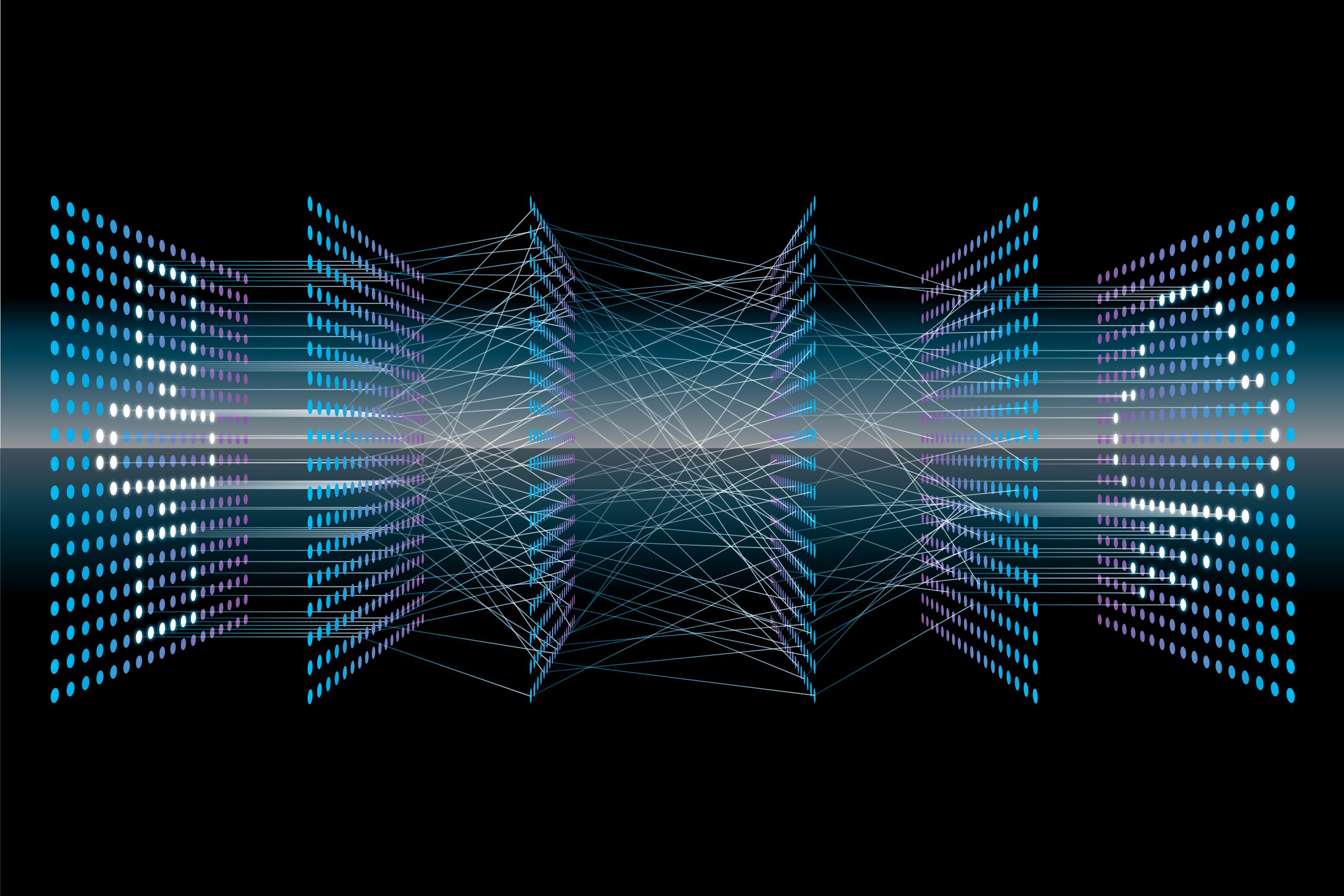
The machine learning team at ECMWF works across all our activities, and includes specialists in machine learning (ML) as well as experts in physical sciences who have trained in machine learning.
It is this mix of backgrounds that forms the expertise and talent of the team.
Some of the team introduce themselves below.
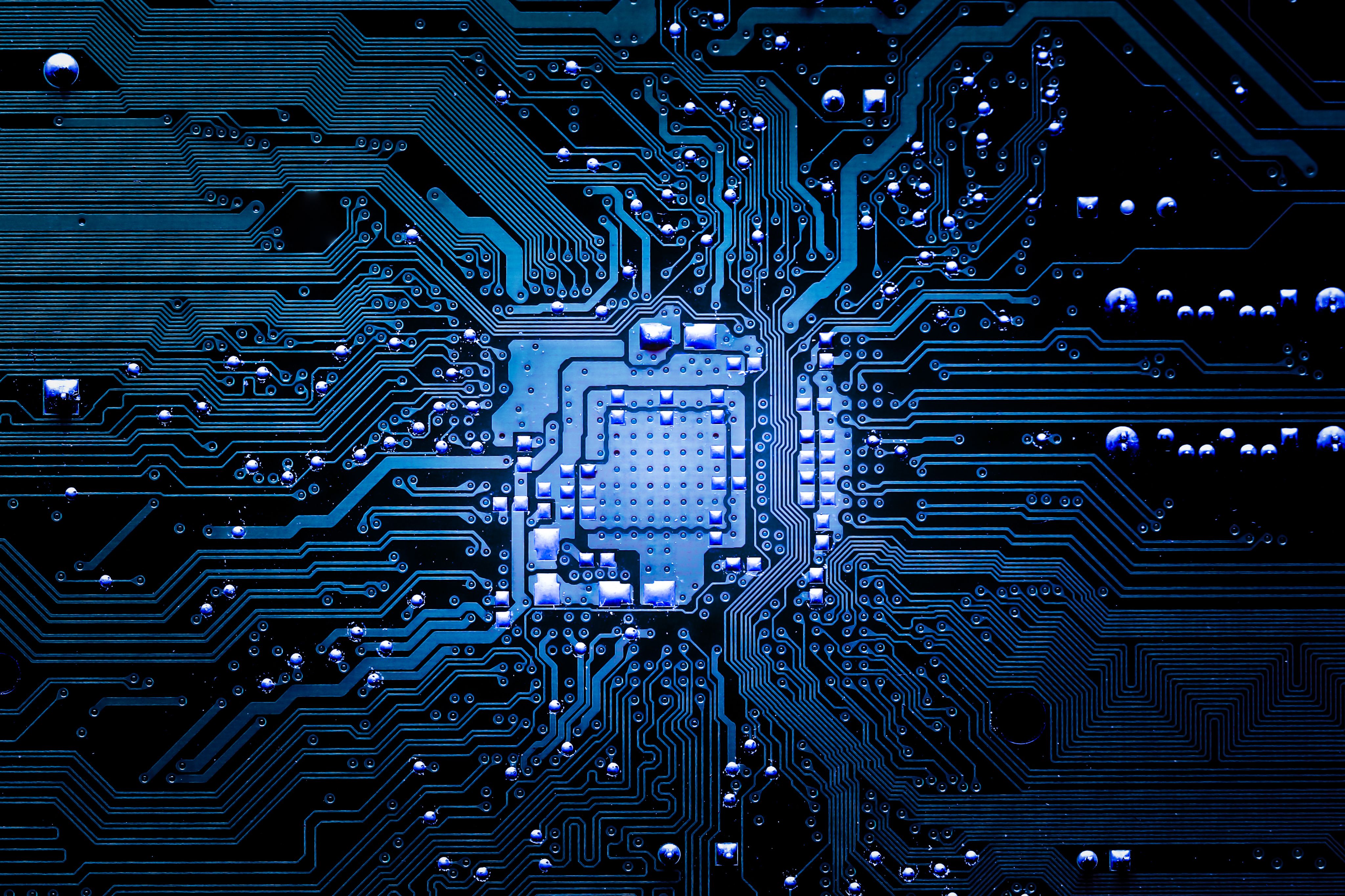
Meet some of the ML team
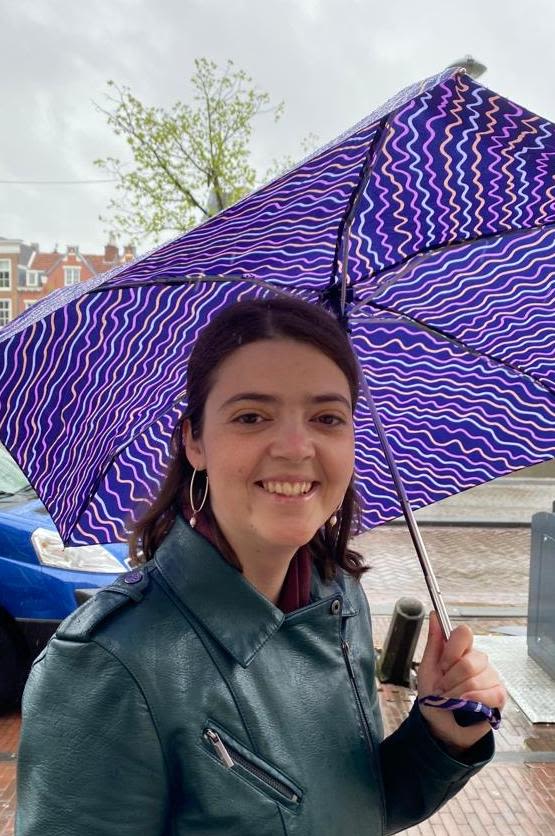
Mariana Clare
The world of machine learning and weather forecasting is constantly evolving and changing, and that makes it a very exciting and stimulating field to be a part of. You never stop learning!
I really enjoy feeling like I’m at the cutting edge of research and that the developments that the team are working on could result in real improvements to how we do weather forecasting.
My role as a 'Scientist for Machine Learning' is focused on adding uncertainty information to the weather forecasts made by our new machine learning forecasting model, called AIFS. As anyone who has planned a summer BBQ knows, weather forecasting is very uncertain and so a good forecast should also be able to predict this uncertainty.
If you want to be part of the new wave of machine learning, working with experts in both machine learning and meteorology, in a place where your work will have a real-world impact, the team at ECMWF is the team for you!
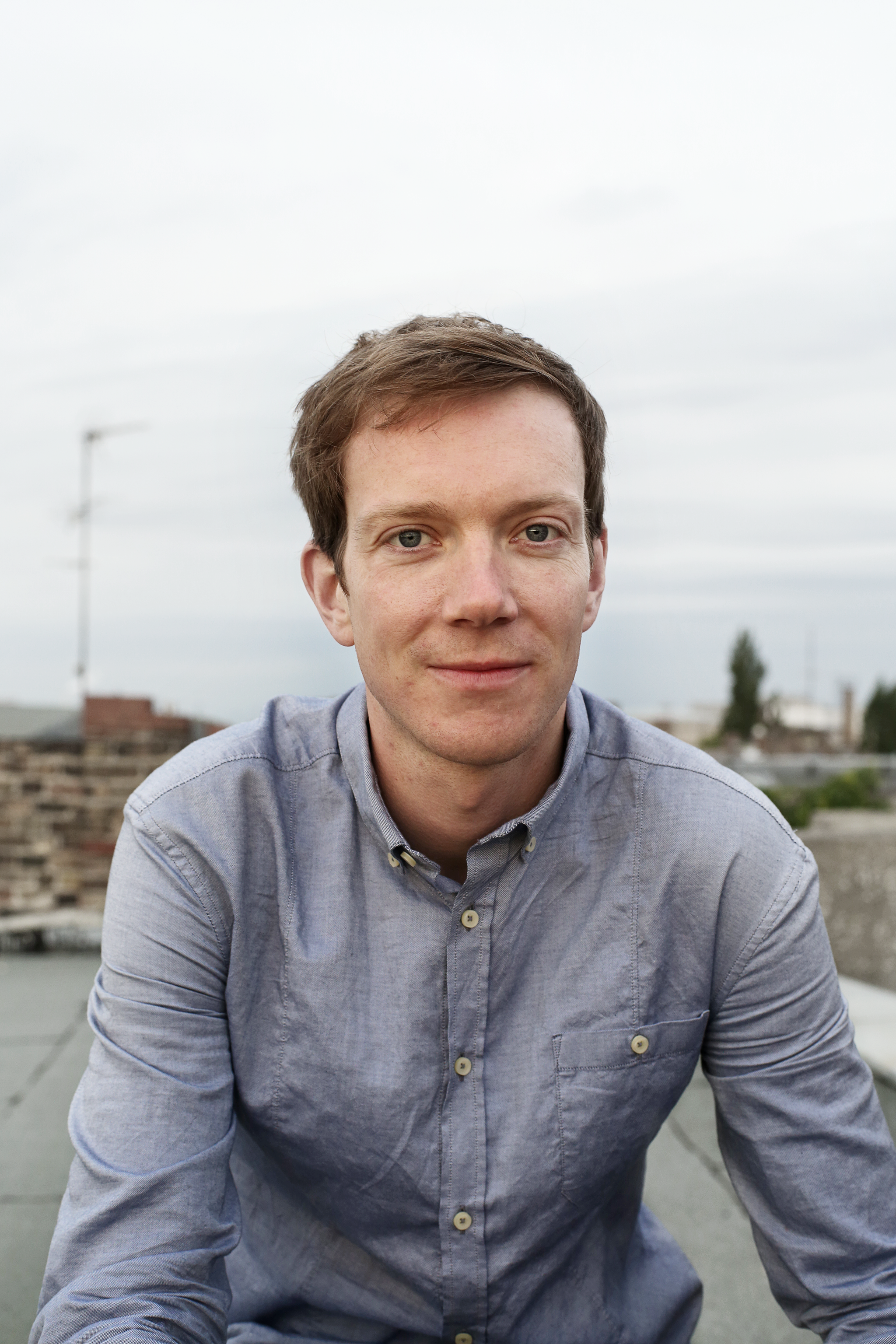
Christian Lessig
I was attracted to work in machine learning as it is a new tool that potentially allows us to solve some of the problems in weather and climate that are difficult to address with conventional approaches.
ECMWF has many brilliant people and great resources, which makes it an exciting place to work at.
I am a senior scientist in the research department, exploring what can be learned from observations and the combination of many different datasets, including simulations and observations.
Working at ECMWF gives you the opportunity to carry out cutting-edge research but also have a real-world impact.
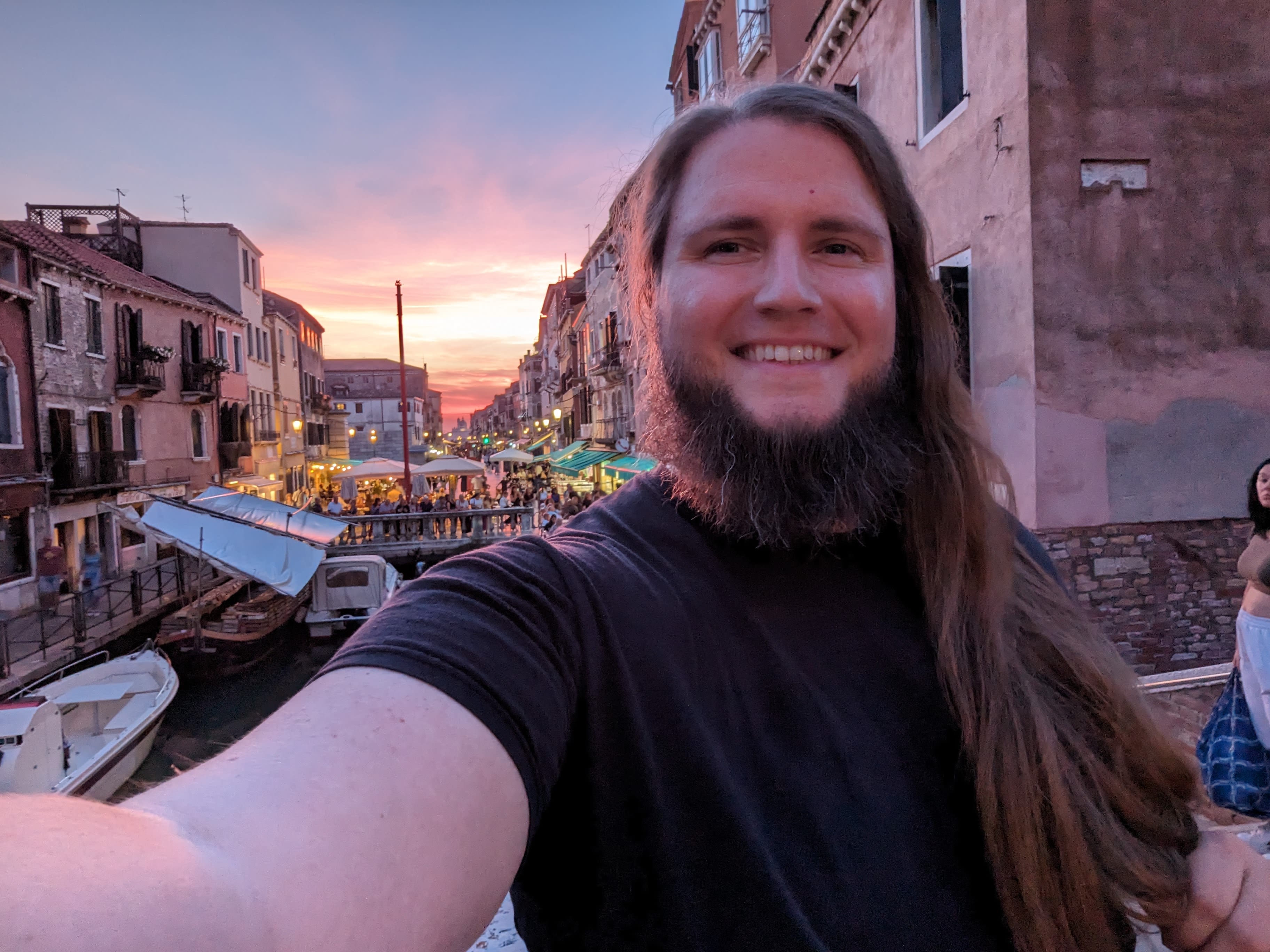
Jesper Dramsch (they/them)
Machine learning was this paradigm shift in 'what a computer can do', from strict logic rules to fuzzy pattern matching. I wanted to be part of this move towards building the new future. Turns out this way of thinking matches my brain very well!
Working on a new paradigm of weather models is extremely fulfilling, and there’s always something interesting and challenging to do. But it can be overwhelming as the machine learning research field never seems to sleep.
My job title is 'Scientist for Machine Learning'. Within that role, I get to do many different things, from data wrangling to ensembles to operationalisation of 'AIFS', our fully data-driven numerical weather prediction model.
Anyone interested in joining our team will be asked a lot of questions to help us find great colleagues who make up our capable and kind group today. I wrote about my hiring experience in detail on my personal webpage to help new applicants!
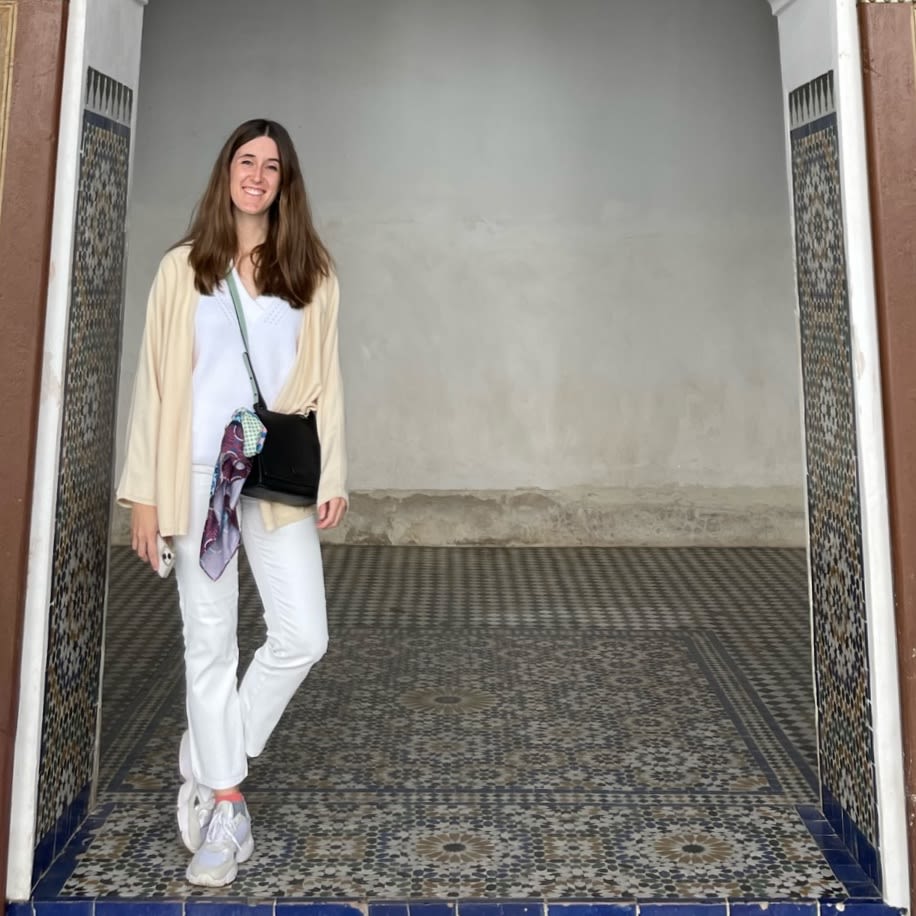
Ana Prieto Nemesio
The opportunity to work with multi-disciplinary teams to address challenges that can ultimately have an impact on our community and society brought me to work on machine learning.
I joined ECMWF quite recently, so I feel like there are many opportunities to learn new things, but there is always someone there to help you!
As a 'Scientist for Machine Learning', I work on two European projects, called MAELSTROM and RODEO, that look at developing machine learning benchmark datasets for weather and climate applications.
I'd bet that someone thinking of applying to join the team wouldn't regret it! It's a team where you will find opportunities to contribute your skills while learning and growing in your career.
Discover more about ML at ECMWF
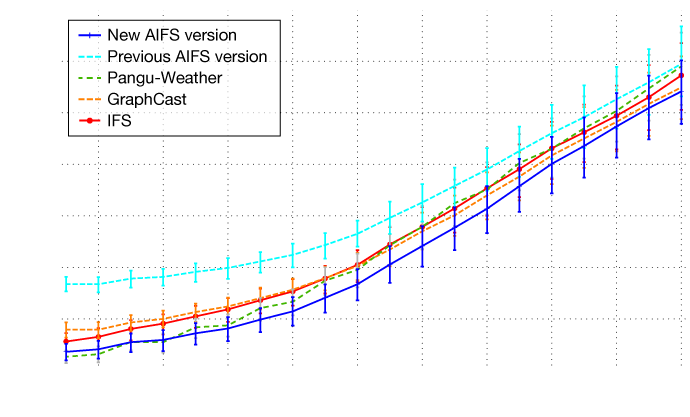
Root-mean-square error for 2 m temperature in the northern hemisphere (top) and southern hemisphere (bottom) measured against synoptic observations for the September–October–November period of 2023. The upgraded AIFS is significantly improved over the previous version, particularly due to the increased spatial resolution.
Root-mean-square error for 2 m temperature in the northern hemisphere (top) and southern hemisphere (bottom) measured against synoptic observations for the September–October–November period of 2023. The upgraded AIFS is significantly improved over the previous version, particularly due to the increased spatial resolution.
AIFS Blog:
ECMWF's Artificial Intelligence/Integrated Forecasting System
On 10 January 2024, we introduced a new version of the AIFS. While the previous version had a spatial resolution of 111 km (~1°), the revised AIFS version has a resolution of 30 km (~0.25°). Its input and output grids are now the native ERA5 reduced Gaussian grid, which provides near-constant resolution across the globe.
There were also architectural changes. The first implementation of the AIFS was built upon Deepmind’s GraphCast approach, based on message-passing graph neural networks and with an internal icosahedral grid with multi-scale edges.
In this new version, the encoder and decoder use attention-based graph neural networks, very similar to a transformer architecture.
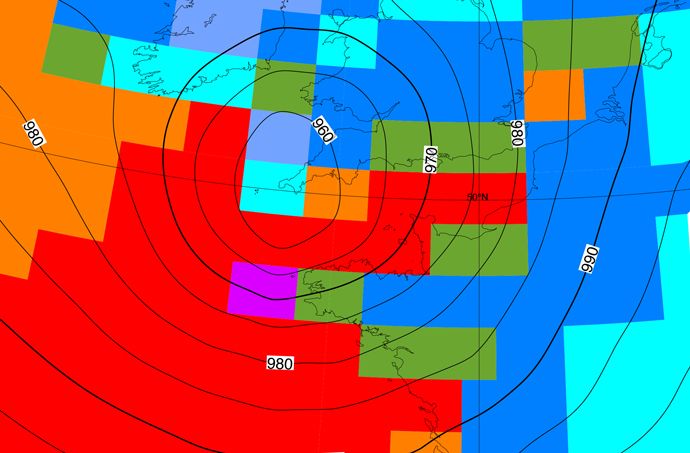
Extract from a 48-hour forecast from ECMWF’s experimental AIFS machine-learning model for mean sea-level pressure and wind speed for 00 UTC on 2 November 2023 (Storm Ciarán).
Extract from a 48-hour forecast from ECMWF’s experimental AIFS machine-learning model for mean sea-level pressure and wind speed for 00 UTC on 2 November 2023 (Storm Ciarán).
Article:
European met services pool resources to intensify use of AI in weather prediction
Over the past two ECMWF Council sessions, in June and December 2023, ECMWF’s Member States have approved two major initiatives aimed at intensifying the development, testing and implementation of machine learning across their weather forecasting chains.
The project approved in June looks specifically at increasing resources within ECMWF to broaden the scope of its work on machine learning (ML), from intensifying ongoing work on a hybrid model mixing conventional and ML methodologies, to developing a fully data-driven forecasting system.
The project approved in December takes an approach which will see the Centre’s Member States join forces to handle aspects of ML applied to weather prediction where they themselves have most expertise. The two complementary projects, running side by side and in full collaboration, recognise the potential of machine learning in weather prediction and build upon substantial activities in this field both at ECMWF and within European meteorological services.
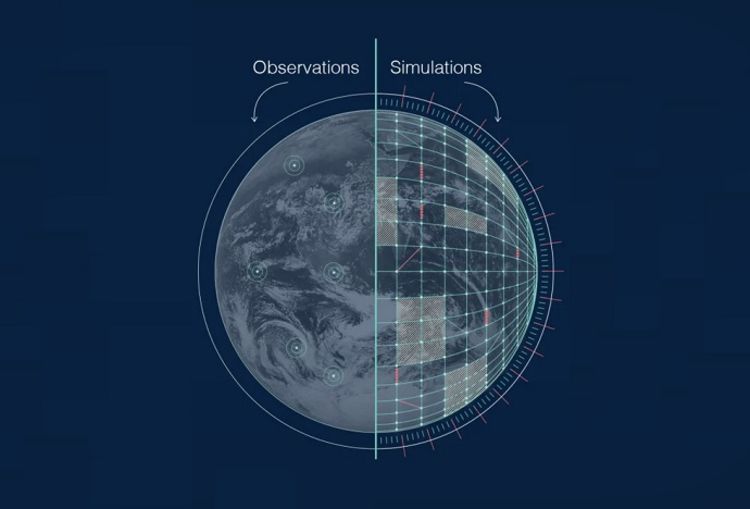
Destination Earth (DestinE) is a visionary initiative to create Digital Twins of the Earth system supporting climate change adaptation policies and decision-making for reducing the impacts of extremes.
Destination Earth (DestinE) is a visionary initiative to create Digital Twins of the Earth system supporting climate change adaptation policies and decision-making for reducing the impacts of extremes.
News:
Phase Two of Destination Earth confirmed
Funding for the second phase of Destination Earth will also intensify the use of recent game changing breakthroughs in machine learning and artificial intelligence across the initiative.
In particular, ECMWF will lead developments towards a machine learning based earth-system model that will support quantifying uncertainties of the earth system digital twins and enhancing their interactivity capabilities.
These developments will allow Destination Earth to become a unique provider on which European industry can test and build new AI-powered products and services. With European values and transparency at its heart it will be a powerful demonstrator on how responsible AI decision processes can be built.

© Copyright 2024
European Centre for Medium-Range Weather Forecasts, Shinfield Park, Reading, RG2 9AX, UK
The content of this web page, excluding images representing individuals, is available for use under a Creative Commons Attribution 4.0 International Public License. See the terms at https://creativecommons.org/licenses/by/4.0/
To request permission to use images representing individuals, please contact pressoffice@ecmwf.int
The information within this web page is given in good faith and considered to be true, but ECMWF accepts no liability for error or omission or for loss or damage arising from its use.